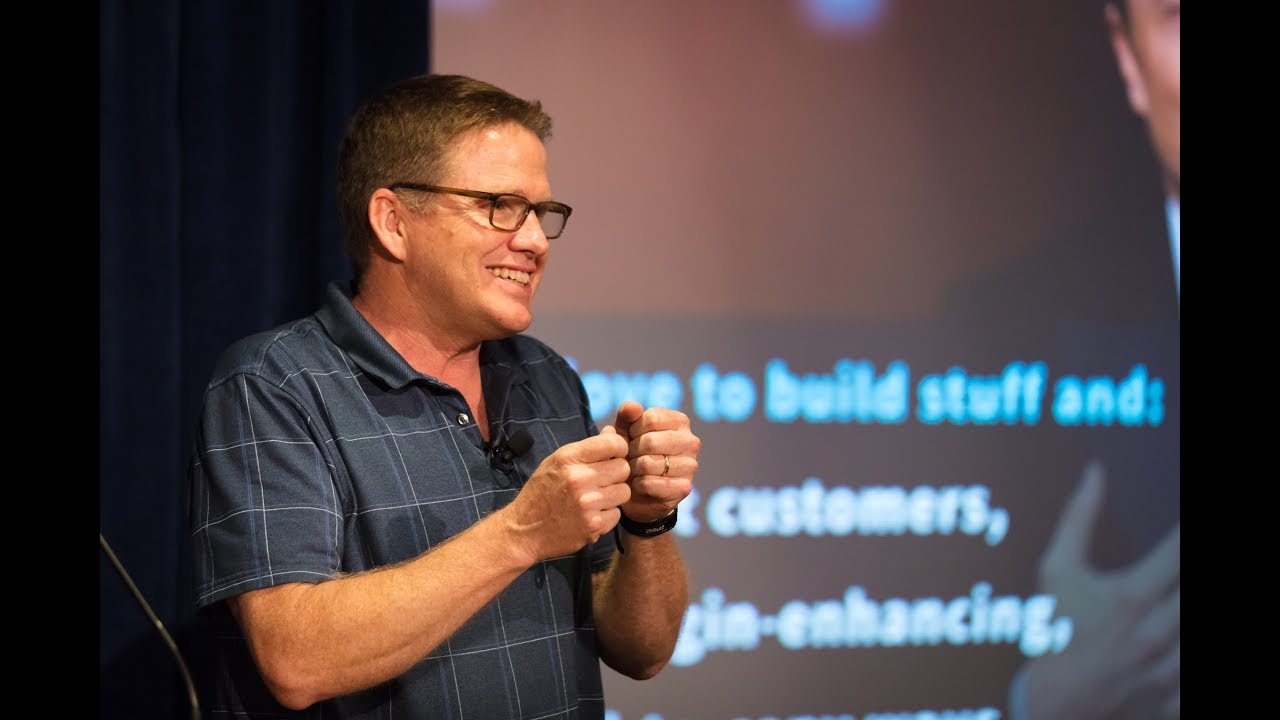
The Strategy-Metric-Tactic Lockup
Gibson: In 2005, Netflix explored six key product strategies. For each strategy, we had a team focused on experiments to prove or disprove each theory. Here's the 2005 high-level product strategy for Netflix, coupled with metrics and projects.
Personalization was the high-level strategy, and the proxy metric for this was the percentage of members who rate at least 50 movies, 5-0, by the end of six weeks. Some key projects, something called the Ratings Wizard and experimenting with the star widget, which is what enabled ratings.
Product strategy number two was keeping things easy and simple. The proxy metric for this was the percentage of members who added at least three titles to their queue in their very first session. That was the minimum number required to create a list that Netflix could send the movies to you. An example project, we worked forever on just simplifying that day one experience.
The strategy number three was social, and for this the proxy metric was the percentage of members who connect to at least one member within Netflix, and the project or tactics was building out this friends network.
Margin enhancement. We measured this in gross profit per member, and some example projects were used DVD sales, our advertising experiments, and then lots of price and plan testing.
Strategy number five, unique movie finding tools. The percentage of members who add at least one title a month via previews. That was our proxy metric. Then example projects, we did personalized previews and we did previews on the synopses on the movie display page.
Then the sixth product strategy we explored in the year 2005 was next-day delivery of DVDs. The proxy metric for this was the percentage of first choice discs delivered the next day. A substantial project or tactic against this strategy was automated hub expansion.
Each of these strategies had a clear proxy metric to determine if the high-level product strategy delivered or not, and there were typically two or three projects, think of these as tactics, that worked to deliver against the strategy. In retrospect, we know that four of the high-level product strategies worked, and two failed. Both social and movie finding tools failed. Over the years, we learned to double down in areas where we moved our proxy metrics and demonstrated retention improvements. We also learned to cut our losses when projects didn't deliver.
Product strategy exercise number five. This is the exercise designed for this article. Using the Netflix Product Strategy as a guide, articulate each of your high-level strategies, the proxy metric for that strategy, along with projects against each strategy
In the next essay, I dive deeper into identifying proxy metrics. I will focus on the theory that a simpler product experience improves retention. Keep listening to learn more.
Suzanne: Can you say more about what makes a good metric? You use the term, to determine if the strategy is delivered. I'm always thinking about the ways in which we define a metric can make them inherently successful or not. How do you know that it's a good metric for your product strategy?
Gibson: The first is to make sure everyone agrees on the high level metric. At Netflix that was retention. No one ever questioned or challenged that. It was a great way of determining is the product getting better? We measured that at the beginning. Retention at Netflix was stinky. 10% would cancel every month. Today it's 2%. When I was there it was like 4.5% would cancel each month.
The challenge is that that's a high level metric that's very hard to move. It's hard to do big A/B tests so you need the proxy. The big surprise to most is that often it took 6, 9, or 12 months to isolate that meaningful proxy. If you were a product leader in streaming, Brandt Avery launched streaming in January 2007. He worked for me.
His proxy metric was the percentage of members that streamed at least 15 minutes in a month. That, we could find the data. We were specific about the 15 minutes because that was the smallest increment of value, the shortest episode we had on TV was 15 minutes. Then our guess was if we could drive that metric north it would actually improve retention, the two would correlate. All of those things turned out to be true but it took us a while to isolate that metric.
Then if you talk to Netflix today I'm sure it's no longer 15 minutes. I'm sure they're looking at percentage of members that are watching at least 20 hours in a month, or 30, or 40 because that's probably the primary driver of retention today. This one is complicated. This one takes time. It takes digging in the dirt. You got to be able to find the data. You got to see if it correlates to retention. The long- term sometimes you want to prove that it actually did move retention via causation.
As much as earlier I tried to simplify the strategy, you have to do a lot of heavy thinking and give time to isolating the right proxy metrics. Because if you get it wrong you could be wasting a lot of time.
Suzanne: When you say it could take 12 months, I mean that implies that there's a lead time in the planning itself because if I follow your framework as you've outlined it in this series you say, "Okay, first we bundle up all of our strategies." Then we kind of cross reference them to say, "Can we make this true against delight? Can we make this true against hard to copy? Can we make this true against margin enhancing." If yes, proceed to how will we know it's successful? Which is the proxy metric. But if you're saying it takes that long to extrapolate or distill down to the measurable thing, what if again I'm at the beginning? What if I need to roll now?
Gibson: That's where I would start with your hypothesis about the right proxy metric. Over time with more information you may choose to vary it.
Suzanne: Okay. It's okay to change on the success signal?
Gibson: Yeah. It is. It is and that happens from time to time.
Suzanne: One of the things that I love about this show, or love to do on the show, is help all of our product people listening in to feel a little bit less alone in that they're not doing things as well as they think everybody else is doing them. This is a little bit of a reversal but I read your essay so many times, and I've followed the worksheets, and I've done the things, and I inevitably find myself coming against the, this is harder to practice than in reality. This is a note to our listeners to say, and this is why we're having these conversations, these are great practicable plans for how to be a great product leader and it's work.
Gibson: Yes. Yes. It is hard. Developing consumer insight is hard. Startups are hard. What you got is the ability to experiment, and with every experiment learn something and apply that learning to the next thing. I know people think Netflix is great, or I hope they do, and a large success. But at the end of the day we got it wrong half the time. We just had high cadence experimentation, and from each of those failed experiments we learned a lot.
Suzanne: You've been listening to episode three with Gib Biddle.
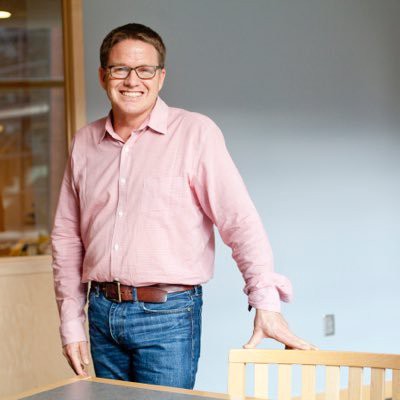
Product strategy answers the question, “How will your product delight customers, in hard to copy, margin-enhancing ways?”
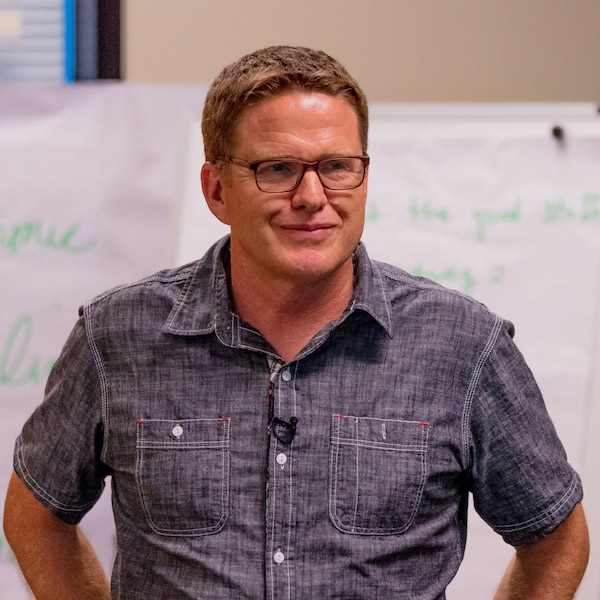
You have ideas to delight customers, to build hard to copy advantage and experiment with your business model. Now what?
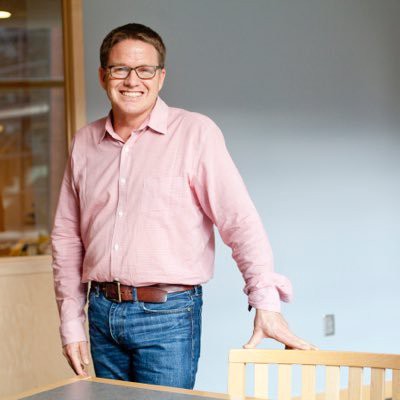
How to define a metric to prove or disprove your hypotheses and measure progress.