Gibson: At Netflix, the metric we used to evaluate overall product quality was monthly retention. This high-level product metric improved significantly over 20 years. In the early days, about 10% of members canceled each month. In 2005, the monthly cancel rate was around 4.5%. Today, it's close to 2%. That is to say only 2% cancel each month.
Using retention as a metric for all projects isn't feasible, however. It's a hard metric to move, and proving a retention improvement requires large-scale A/B tests. Lower-level metrics, or proxy metrics, are easier and faster to move than a high-level metric like retention. Ideally, moving a proxy will improve the high-level metric. In the case of Netflix, that is retention, and there is a correlation between the proxy and the high-level metric. Later, you can prove causation via an A/B test.
How do you measure simple? One of our hypotheses was that a simpler member experience would improve retention, but how do you measure simple, and how do you demonstrate that it improves retention?
We began by exploring customer service data. Why do members call or email Netflix with questions or complaints? What links do they click on when they visit the help pages? Where do customers get confused? Over time, we focused our efforts on new members as a large number of potential customers at the top of the sign-up funnel provided a substantial business opportunity.
We talked to new members in one-on-one sessions and focus groups. We asked a small group of customers to write a journal describing their weekly activity with Netflix. Last, we looked at existing data for the new member sign-up flow, as well as their first few weeks with the service.
One point of confusion among new members, our early DVD-by-mail service required customers to create an ordered list of movies that we would send to them, but some new members failed to add any videos to their Netflix queue. Some new members chose a plan, entered their credit card information, then asked, “Now what?” The notion of adding at least three titles to their queue confused many new members.
It was clear we needed to simplify the sign-up process and make it easier for customers to create a list of movies. Eventually, we executed a series of day one projects focused on eliminating steps, reducing cognitive overhead, and providing clarity about how the service worked.
The proxy metric we devised was the percentage of new members who add at least three titles to their queue during their first session. When we first looked at the data, 70% of new members added at least three titles to their queue during their first session. By the end of the year, after a series of fast-paced experiments, we increased this percentage to 90%.
Over the same period, we drove month one retention from 88% to 90%. Both retention and our simple metric moved together. We chose not to take the time, however, to execute a large-scale A/B test, because we were confident that the more straightforward experience improved retention.
The right proxy metric. Proxy metrics are a stand-in for your high-level product metric. First, you seek a correlation between your high-level metric and the proxy metric. Later you work to prove causation.
Here's a simple model to define proxy metrics. The percentage of, fill in the blank, members, new customers, returning customers, who do at least, insert the minimum threshold for user action, by X period in time.
Some examples of proxies for retention at Netflix. Percent of members who add at least one member to their friends list within six months. The Netflix friends feature launched with 1% of members using the feature, grew to 6% over three years, then Netflix killed the feature. The assumption was that the friends proxy metric needed to surpass 20% to achieve a meaningful retention improvement, and we were nowhere close to that 20%.
Percent of members who stream at least 15 minutes of video in a month. At the launch of watch instantly in 2007, this metric was 5%. Today, it's north of 90%. We chose 15 minutes because it was the smallest increment of value. The shortest TV episode was 15 minutes. I'm sure Netflix measures a similar proxy today but at a variety of much higher hurdles, likely the percent of members who watch at least 10, 20, 30 hours a month.
Percent of members who add at least six DVDs to their queue in a month. The merchandising team's job was to make it easy for members to find and add movies to their list. Initially, the metric was 70%. With effort, we moved it to 90%.
Percent of new members who rate at least 50 movies in their first six weeks with the service. This metric was our proxy for our personalization efforts. The theory was that if customers were willing to rate movies, they valued the movie recommendations Netflix provided. We drove this metric from the low single digits into the high 20s over a few years.
Percent of first choice DVDs delivered to members the next day in the mail. One of the early insights about our DVD-by-mail service was that delivering the first choice DVD the next day was critical. At first measurement, the metric was 70%. We drove this metric to 90% by standing up 50 automated DVD delivery hubs throughout the US. We also integrated the inventory data from each delivery hub with the merchandising system. We only merchandised titles that were unavailable in a member's local shipping center.
As you evaluate potential metrics, make sure the proxy does the following. It's measurable. You can find, collect, and measure the data. Ideally, you assess the metric in an A/B test, and the metric helps answer the question, should we launch this feature or not? In evaluating a new product strategy, ask yourself, in an A/B test, what metric would we use to make a go/no-go decision?
Is moveable. You can affect the metric through changes to the product experience. It's important that your proxy metric can move.
It's important that your proxy metric does not state an average. The danger of averages is you may move the metric by inspiring a small subset of customers to do a lot more of something, but this may not affect enough members to improve the overall product experience.
Your proxy metric correlates to your top line high-level metric. For Netflix, successful proxy metrics and retention moved together. Long-term, you hope to prove causation via a large-scale A/B test.
It's good if your proxy metric specifies new versus existing members. As Netflix grew, we learned to focus our effort on new members. We believed that to become a sizeable worldwide service, we needed to optimize for new members. We would test features with new members, then roll out to all members based on positive results. Existing members sometimes noticed the change, complained about it, but rarely canceled. Occasionally, if we believed there was a real risk of hurting retention, we ran an A/B test with existing members, too.
Last, it's important that your proxy metric is not gameable. One product manager focused on customer service. His job was to make it easy for members to help themselves and not call our customer service team via our 800 number. The metric that defined his role was contacts per 1,000 customers, and the goal was to lower this metric below 20 contacts per 1,000 customers. But he quickly discovered he could game the metric by hiding the 800 number. Consequently, we revised the proxy, contacts per 1,000 members with the 800 number available within two clicks.
A big surprise at Netflix, we made decisions quickly, but isolating the right proxy metric sometimes took six months. It took time to capture the data, to discover if we could move the metric, and to see if there was causation between the proxy and retention. Given a trade-off of speed and finding the right metric, we focused on the latter. It's costly to have a team focused on the wrong metric. Eventually, each of the product managers on my team could measure their performance through one or two proxy metrics that contributed to improving monthly retention.
Product strategy exercise number six. For this, based on this essay, identify your North Star metric, your high-level metric equivalent to Netflix's retention. Now, re-look at your work from the last essay, The Strategy/Metric/Tactic Lockup, and re-evaluate your proxy metric for each high-level strategy against this coaching for what constitutes a good proxy metric.
My next essay outlines an alternative approach to defining your product strategy. It's called Working Top-Down and Bottom-Up.
Suzanne: In our last conversation I was challenging this notion of a good metric and I think you kind of spoke to this before but it's so relevant in this essay in particular. You set up the difference between a north star metric, a sort of big metric, and then the proxy metric. Can you explain a little bit more for our listeners how these concepts relate within your framework?
Gibson: Imagine you're the product leader at a company. That's your job, and your job is to make the product better. That's it. What metric would you land on to define what better means? Generally speaking that's an engagement metric, that a product that does a better job of engaging your customers likely is a better product. Oftentimes companies will measure monthly active users. What percentage of folks came back at least month to month. At Netflix we had monthly retention.
Anyways. That's the high level metric. I've learned recently not to call that a north star metric. It creates some confusion; I'll call it the high level engagement metric. How do you know if your product's good or bad? What metric would you look for? Then if I layered down... I love to create organizations where each of my product leaders has a swim lane that they own. They can actually define their product metric.
I had retention at Netflix. They could define the one proxy that they're going to move. This is a joke if people understand me well say, "Well, I'm going to kill you," but it gave this sort of radical job clarity right? High level metric, proxy metric for each strategy, or a product leader in a swim lane. Then each of them could define strategy in their area.
These things don't map tightly. Netflix, at a high level we knew strategy was important. In fact we dedicated a whole swim lane to personalization. In other areas it can't quite map the organization against the strategy. There's some stuff you have to work out. Anyways, for me the job's a little complicated.
Organizations are complicated. I kept reinforcing at the high level what the product strategies were, the metrics, the tactics for projects. Then I depended on each of the product leaders in their swim lanes to do the same. Sometimes you could sort of see the relationships among them and sometimes you can't. It's okay that things are a little messy and dirty.
Suzanne: How then do you know when and if your metric was wrong, but maybe the experiment was successful?
Gibson: Whoa. I'm trying to think if I ever had that. A successful experiment? No. The first thing I was thinking of - we knew it was a failed experiment because we couldn't move the proxy metric. That's how we knew. A good example of that was our social effort. It was called Friends. The proxy was the percentage of Netflix members that created a connection with at least one friend within Netflix.
We drove that up to, maybe we got up to 6% which wasn't big enough. If you're trying to move retention you need something like 20% of your members engaged in that. That was an example of how powerful and helpful the proxy metric was to evaluate progress and identify the fact we hadn't made enough. All right. Have I ever seen an instance where we had a success-
Suzanne: Well, and not even that you had a success. I guess what I'm really hinting at is if you don't set the right metric is it possible then that you're only seeing the success of the experiment through that lens and what if that's the wrong lens for evaluating the merit of the strategy itself?
Gibson: Yeah. Well, what if you're evaluating the wrong metric? You've got a proxy metric that's wrong. Everything looks good and then you're realizing you're not really creating customer or shareholder value.
Suzanne: You've been listening to musings on essay number four.
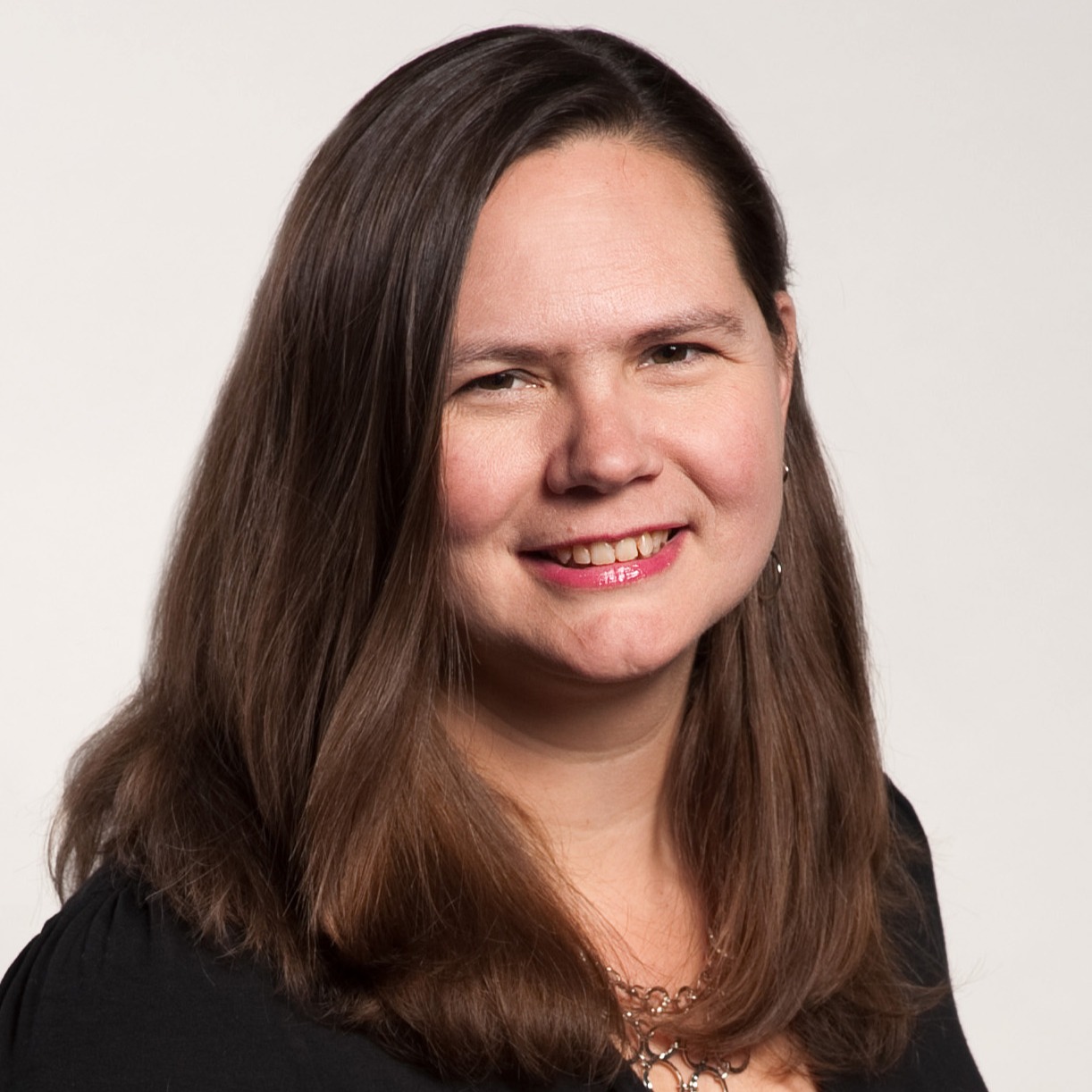
In this episode:
- Where do startups go wrong with implementing OKRs
- Can OKRs really scale for enterprise?
- What are pipelines and how do they change the way we think about product roadmaps?
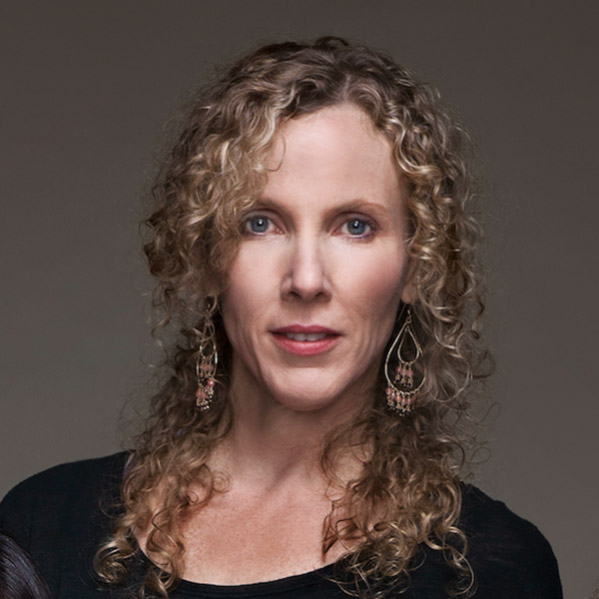
In this episode:
- From retail to product management
- Why relationship building is the number one required skill a product manager could have
- The value of having confidence with humility
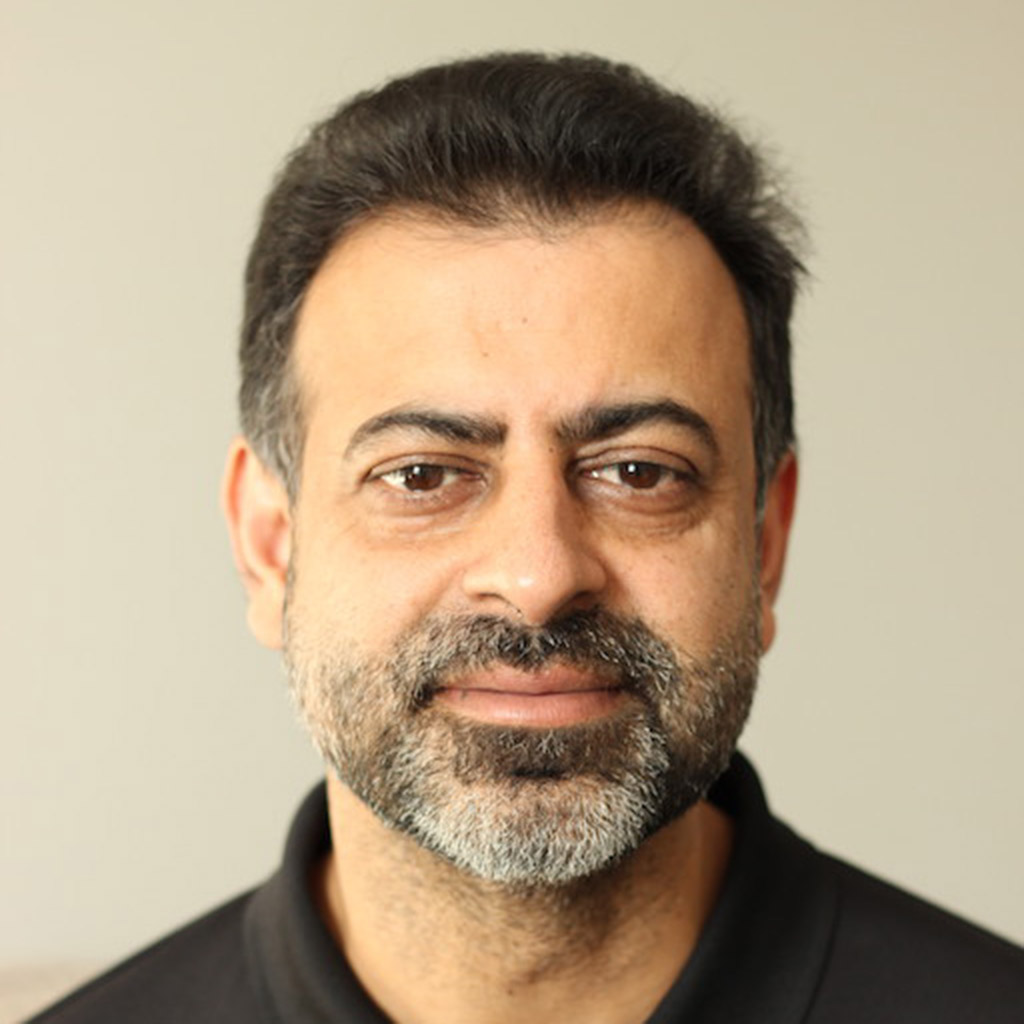
In this episode:
- Establishing a clear vision of your career path
- Using metrics to answer burning product questions
- What product managers can learn from biology